Data Management
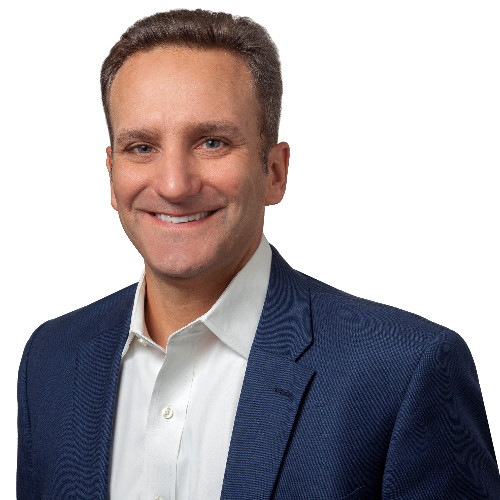
Neal Sivie
CTO | Partner
Why should corporate real estate teams care about data management?
Corporate Real Estate (CRE) will always be a blend of art and science. The new information landscape requires big data and the insights it brings so that you can focus on strategy and making data-driven and solution-driven recommendations. To get there, companies need a data infrastructure strategy.
CRE data empowers leaders to modify strategy in a rapidly evolving market. Moving to a predictive model, informed by performance and real-time data in leasing and asset management will improve your team’s ability to make decisions in the present based on future conditions.
Value of Data Management
In a recent survey of Australian CEOs, KPMG found that 84% of CEOs are accelerating digital transformation following COVID-19.(1)
Data Management is the digital fuel for digital transformation. This includes how data systems communicate with each other to provide data insight and business intelligence. The transparency and neutrality of the process are critical for an organization to obtain their goals and achieve increased productivity, differentiation, and continuous innovation.
Real Estate, Facilities, and Property management providers are responsible for the discrete systems they use to execute on behalf of their customers and stakeholders. This siloed approach provides reporting but is limited in the delivery of enterprise-level business intelligence and predictive analytics. For example, you may have insights into how a particular piece of equipment is running based on the work order history and sensor level data, however, you will not be able to connect this with other insights beyond what is managed by one supplier.
A periodic review of a data infrastructure strategy will help to validate the current-state business requirements, the utility of the data, and will identify opportunities to better align across operations and the new information landscape. This assessment should: include a deeper dive on how data is originated and is exchanged across properties;, assess providers and portfolios; identify data gaps and silos; deploy tools and applications to close gaps; and validate Data Governance standards to ensure that data is clean, structured, and secure for analysis.
Data Management Program
1. Data Governance: A process of planning and managing data, its availability, integrity, usability, the standards that affect its accuracy, the security of data, and the policy that controls its usage. Data ownership and accountability for the data must be clearly defined for the governance process to be effective.
2. Data Architecture and Modeling: The architecture process determines the overall structure of the data, identifying what data is collected, how it is stored, managed, and used. Modeling enables an organization to find, analyze, understand how data interoperate.
3. Storage: Database: Organized data into tables by rows and columns. These are best suited for CRE groups to structure transactional and sensor data.
- Big Data: A composition of structured and unstructured data, static data, streaming (dynamic) data, and histories.
4. Business Intelligence and Machine Learning Enablement:
- Data integration platforms: The most used integration is Extract, Transform, and Load (ETL). The process pulls data from various information systems, converts the data to a desired format, and then loads the data into a staging area for data quality review, compliance, and structure.
- Data Warehouse: Structured data from systems that enable analytics, business intelligence, and enterprise reporting.
- Data Lake: Combines big data and a stored pool of unstructured data from various systems which can support predictive analytics and machine learning.
References
KPMG (2020), Global CEO Outlook 2020: COVID-19…Australian CEOs are reorganizing their priorities; https://home.kpmg/au/en/home/insights/2020/09/global-ceo-outlook-2020.html